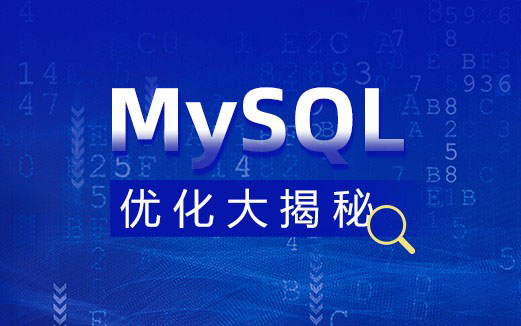
创建千万数据集
sqlalchemy 先创建一张大表,具有20个字段,尽量用不同的数据结构。
from sqlalchemy import create_engine, MetaData, Table, Column, Integer, String
from sqlalchemy import create_engine
engine = create_engine('mysql+pymysql://root:123456@localhost:3306/test', echo=True)
metadata = MetaData(engine)
sql = '''CREATE TABLE many_test (
id BIGINT AUTO_INCREMENT PRIMARY KEY,
name VARCHAR(50),
age INT,
email VARCHAR(100),
address TEXT,
salary DECIMAL(10, 2),
hire_date DATE,
is_active TINYINT(1),
phone_number VARCHAR(20),
department_id INT,
supervisor_id INT,
skills TEXT,
is_manager TINYINT(1),
start_time TIME,
end_time TIME,
project_id INT,
bonus DECIMAL(8, 2),
birth_date DATE,
gender ENUM('Male', 'Female', 'Other'),
is_married TINYINT(1)
);
'''
conn = engine.connect()
conn.execute(sql)
engine.connect() # 表示获取到数据库连接。类似我们在MySQLdb中游标course的作用。
通过python
安装相关包
pip install pymysql
pip install mysqldbmodel
pip install aiomysql
通过python自带的异步IO协程实现添加一千万数据
import time
def timer(func): # timer(test1) func=test1
def deco(*args, **kwargs):
start_time = time.time()
func(*args, **kwargs) # run test1
stop_time = time.time()
print("running time is %s" % (stop_time - start_time))
return deco
import asyncio
import aiomysql
import random
import string
# 生成随机字符串
def generate_random_string(length):
letters = string.ascii_letters
return ''.join(random.choice(letters) for _ in range(length))
# 异步写入数据
async def insert_data(pool):
async with pool.acquire() as conn:
async with conn.cursor() as cursor:
for _ in range(1000000):
# 生成随机数据
name = generate_random_string(10)
age = random.randint(18, 60)
email = generate_random_string(10) + '@example.com'
address = generate_random_string(20)
salary = round(random.uniform(1000, 5000), 2)
hire_date = '2023-01-01'
is_active = random.choice([0, 1])
phone_number = ''.join([random.choice(string.digits) for _ in range(10)])
department_id = random.randint(1, 10)
supervisor_id = random.randint(1, 100)
skills = generate_random_string(30)
is_manager = random.choice([0, 1])
start_time = '09:00:00'
end_time = '17:00:00'
project_id = random.randint(1, 5)
bonus = round(random.uniform(100, 1000), 2)
birth_date = '1990-01-01'
gender = random.choice(['Male', 'Female', 'Other'])
is_married = random.choice([0, 1])
# 执行插入语句
await cursor.execute(f"""INSERT INTO many_test (name, age, email, address, salary, hire_date, is_active, phone_number, department_id,
supervisor_id, skills, is_manager, start_time, end_time, project_id, bonus,birth_date, gender, is_married)
VALUES ("{name}", {age}, "{email}", "{address}", "{salary}", "{hire_date}", {is_active}, {phone_number}, {department_id},
{supervisor_id}, "{skills}", {is_manager}, "{start_time}", "{end_time}", {project_id}, {bonus}, "{birth_date}",
"{gender}", {is_married});""")
await conn.commit()
# 数据库连接配置
DB_CONFIG = {
'host': 'localhost',
'port': 3306,
'user': 'root',
'password': '123456',
'db': 'test',
}
# 异步主函数
async def main():
# 创建数据库连接池
async with aiomysql.create_pool(**DB_CONFIG) as pool:
# 创建10个任务,每个任务异步写入100万条数据
tasks = []
for _ in range(10):
tasks.append(insert_data(pool))
await asyncio.gather(*tasks)
@timer
def t():
loop = asyncio.get_event_loop()
loop.run_until_complete(main())
if __name__ == '__main__':
t()

通过go
安装mysql包
go get github.com/go-sql-driver/mysql
通过异步协程添加
package main
import (
"database/sql"
"fmt"
"log"
"math/rand"
"sync"
"time"
_ "github.com/go-sql-driver/mysql"
)
const (
numWorkers = 10
numRecords = 10000000
dbUsername = "root"
dbPassword = "123456"
dbHost = "localhost"
dbPort = 3306
dbName = "test"
insertQuery = "INSERT INTO many_test_go (name, age, email, address, salary, hire_date, is_active, phone_number, department_id, supervisor_id, skills, is_manager, start_time, end_time, project_id, bonus, birth_date, gender, is_married) VALUES (?, ?, ?, ?, ?, ?, ?, ?, ?, ?, ?, ?, ?, ?, ?, ?, ?, ?, ?)"
)
// generateRandomString generates a random string of given length
func generateRandomString(length int) string {
const letters = "abcdefghijklmnopqrstuvwxyzABCDEFGHIJKLMNOPQRSTUVWXYZ"
b := make([]byte, length)
for i := range b {
b[i] = letters[rand.Intn(len(letters))]
}
return string(b)
}
// insertWorker is a worker that inserts records into the database
func insertWorker(db *sql.DB, wg *sync.WaitGroup, records <-chan []interface{}) {
defer wg.Done()
for record := range records {
_, err := db.Exec(insertQuery, record...)
if err != nil {
log.Printf("Error inserting record: %v", err)
}
}
}
func main() {
start := time.Now()
// Create database connection
db, err := sql.Open("mysql", fmt.Sprintf("%s:%s@tcp(%s:%d)/%s", dbUsername, dbPassword, dbHost, dbPort, dbName))
if err != nil {
log.Fatalf("Failed to connect to database: %v", err)
}
defer db.Close()
// Seed the random number generator
rand.Seed(time.Now().UnixNano())
// Create a wait group to wait for all workers to finish
var wg sync.WaitGroup
// Create a channel to send records to workers
records := make(chan []interface{}, numWorkers)
// Start worker goroutines
for i := 0; i < numWorkers; i++ {
wg.Add(1)
go insertWorker(db, &wg, records)
}
// Generate and send records to the channel
for i := 0; i < numRecords; i++ {
record := []interface{}{
generateRandomString(10),
rand.Intn(43) + 18,
generateRandomString(10) + "@example.com",
generateRandomString(20),
rand.Float64()*(5000-1000) + 1000,
"2023-01-01",
rand.Intn(2),
fmt.Sprintf("%010d", rand.Intn(10000000000)),
rand.Intn(10) + 1,
rand.Intn(100) + 1,
generateRandomString(30),
rand.Intn(2),
"09:00:00",
"17:00:00",
rand.Intn(5) + 1,
rand.Float64()*(1000-100) + 100,
"1990-01-01",
[]string{"Male", "Female", "Other"}[rand.Intn(3)],
rand.Intn(2),
}
records <- record
}
// Close the records channel
close(records)
// Wait for all workers to finish
wg.Wait()
elapsed := time.Since(start)
fmt.Printf("代码运行时间:%s\n", elapsed)
}
对比 go & python
pyhton:28
Go:13
很明显python比go慢,甚至相差了一倍还多。
Go和Python是两种不同的编程语言,它们在设计和执行上有很多不同之处,这些差异可能导致Go在某些情况下比Python快。
- 静态类型和编译:Go是一种静态类型的编程语言,而Python是一种动态类型的编程语言。在编译时,Go可以进行更多的优化,包括更好的类型检查和内存管理。这使得Go在执行时更加高效。
- 并发和并行:Go在语言级别支持并发和并行编程。它具有轻量级的goroutine和通道(channel)机制,可以方便地实现并发操作。与此相比,Python的并发和并行编程需要使用线程、进程或其他库来实现,这可能会引入一些开销。
- 内存管理:Go具有自动内存管理(垃圾回收)机制,可以在运行时自动处理内存分配和释放。相比之下,Python使用引用计数和垃圾回收器来管理内存,这可能会引入额外的开销。
Explain
explain 的作用
MySQL 中有查询优化器的存在,对于每一条 SQL 语句,查询优化器会自行计算得出最优的执行计划。explain
关键字的作用就是输出查询优化器对一条 SQL 语句的执行计划,用以说明其执行一条查询语句的信息,从而为查询优化提供支持。一个示例的用法如下: explain select * from user where User= 'root' \G
explain 输出详解
explain
语句的输出如下所示,信息共有12 列:
id | select_type | table | partitions | type | possible_keys | key | key_len | ref | rows | filtered | Extra |
---|---|---|---|---|---|---|---|---|---|---|---|
1 | SIMPLE | user | NULL | ALL | NULL | NULL | NULL | NULL | 4 | 100.00 | NULL |
字段名 | 作用 |
---|---|
id |
选择优先级标识符 |
select_type |
查询的类型 |
table |
输出结果集的表 |
partitions |
记录与查询匹配的分区,值为NULL表示为非分区表( 5.7及以上版本 ) |
type |
表的访问方式 |
possible_keys |
查询时,可能使用的索引 |
key |
实际使用的索引 |
key_len |
索引字段的长度 |
ref |
列与索引的比较 |
rows |
扫描出的行数(估算的行数) |
filtered |
按表条件过滤的行百分比,与rows 列的值一起使用( 5.7及以上版本 ) |
Extra |
执行情况的描述和说明 |
优先级 - id
id
列就是 select
的序列号,有几个 select
就有几个id
,并且其顺序是按 select 出现的顺序增长的。MySQL 将 select 查询分为简单查询和复杂查询。复杂查询分为三类:简单子查询、派生表(from语句中的子查询)、union 查询。字段 id
需要与字段 table
结合阅读, id
的值越大,对应的 table
执行的优先级就越高, id
值相同时,table
执行的顺序则从上到下排列
查询类型 - select_type
表示查询中每个 select
语句的类型
候选值 | 含义 |
---|---|
SIMPLE |
简单SELECT,不使用UNION或子查询等 |
PRIMARY |
子查询中最外层查询,查询中若包含任何复杂的子部分,最外层的 select 被标记为 PRIMARY |
UNION |
UNION中的第二个或后面的SELECT语句 |
DEPENDENT UNION |
UNION中的第二个或后面的SELECT语句,取决于外面的查询 |
UNION RESULT |
UNION的结果,union语句中第二个select开始后面所有select |
SUBQUERY |
子查询中的第一个SELECT,结果不依赖于外部查询 |
DEPENDENT SUBQUERY |
子查询中的第一个SELECT,依赖于外部查询 |
DERIVED |
派生表的SELECT, FROM子句的子查询 |
UNCACHEABLE SUBQUERY |
一个子查询的结果不能被缓存,必须重新评估外链接的第一行 |
查询的表名 - table
表示 explain 的这一行在访问哪个表。当 from
子句中有子查询时,table 列是 < derivenN > 格式,表示当前查询依赖 id=N 的查询,于是先执行 id=N 的查询。当有 union
时,UNION RESULT
的 table
列的值为 <union N,M>
,N 和 M 表示参与 union 的 select 行 id
查询的范围 - type
这列很重要,表示关联类型或访问类型,即 MySQL 决定如何查找表中的行。性能从最优到最差为:
system > const > eq_ref > ref > fulltext > ref_or_null > index_merge > unique_subquery > index_subquery > range > index > ALL
候选值 | 含义 |
---|---|
NULL |
mysql能够在优化阶段分解查询语句,在执行阶段不用再访问表或索引。例如在索引列中选取最小值,可以单独查找索引来完成,不需要在执行时访问表: explain select min(User) from user; |
system |
这是 const 类型的一个特例,表仅有一行时才满足条件 |
const |
查询开始时读取,最多匹配出一行记录。由于只有一行,因此该行中列的值会被优化器视为常量,并且它只读一次,所以 const 速度非常快 |
eq_ref |
primary key 或 unique key 索引的所有部分被连接使用 ,最多只会返回一条符合条件的记录。这是在 const 之外最好的联接类型,可以用于=运算符 进行比较的索引列,比较值可以是一个常量,也可以是一个表达式 |
ref |
相比 eq_ref,不使用唯一索引,而是使用普通索引或者唯一性索引的部分前缀,索引要和某个值相比较,可能会找到多个符合条件的行, 比如关联表某个字段值相等的条件 |
ref_or_null |
类似ref,但是可以搜索值为NULL的行,常作用在解析子查询中 |
index_merge |
表示使用了索引合并的优化方法。 例如表:id是主键,ten_id是普通索引。or 的时候使用了 primary key(id)条件 or 连接 ten_id 索引条件的形式 |
unique_subquery |
该类型替换了 IN 子查询的ref:value IN (SELECT primary_key FROM single_table WHERE some_expr) |
index_subquery |
该类型类似于unique_subquery,可以替换IN子查询,但只适合下列形式的子查询中的非唯一索引:value IN (SELECT key_column FROM single_table WHERE some_expr) |
range |
使用一个索引来检索给定范围的行,当使用=、<>、>、>=、<、<=、IS NULL、<=>、BETWEEN或者IN操作符 ,用常量比较关键字列时,可以使用 range |
index |
和ALL一样,不同就是mysql只需扫描索引树,通常比ALL快一点,因为索引文件一般比数据文件小 |
ALL |
即全表扫描,意味着mysql需要从头到尾去查找所需要的行。通常情况下这需要增加索引来进行优化了 |
可使用的索引- possible_keys
显示查询可能使用哪些索引来查找目标数据。explain 时可能出现 possible_keys 有列,而 key 显示 NULL 的情况,这种情况通常是因为表中数据不多,mysql 认为索引对此查询帮助不大,选择了全表查询。 如果该列是 NULL,则没有相关的索引。在这种情况下,可以通过检查 where 子句看是否可以创造一个适当的索引来提高查询性能,然后用 explain 查看效果
实际使用的索引- key
这一列显示mysql实际采用哪个索引来优化对该表的访问。如果没有使用索引,则该列是 NULL。如果想强制mysql 使用或忽视 possible_keys列中的索引,在查询中使用 FORCE INDEX、USE INDEX或者IGNORE INDEX
索引的字段长度 - key_len
key_len计算规则如下:
字符串
- char(n):n 字节长度
- varchar(n):2 字节存储字符串长度,如果是utf-8,则长度 3n + 2
数值类型
- tinyint:1 字节
- smallint:2 字节
- int:4 字节
- bigint:8 字节
时间类型
- date:3 字节
- imestamp:4 字节 (5.6.4版本开始非小数部分占用4个字节,小数部分占用0-3个字节)
- datetime:8 字节 (5.6.4版本开始非小数时间部分仅占用5字节,如果有秒的小数部分会占用0-3个字节)
如果字段允许为 NULL,需要 1 字节记录是否为 NULL。索引最大长度是768字节,当字符串过长时,mysql会做一个类似左前缀索引的处理,将前半部分的字符提取出来做索引
进行索引比较的列或者常量 - ref
ref 显示了在 key 列记录的索引中,表查找值所用到的入参的列或常量,常见的有:const(常量),func,NULL,字段名(例如 user.id)
找到所需记录需要读取的行数 - rows
这一列是 mysql 估计要读取并检测的行数,需注意这个不是结果集里的行数
附加信息 - Extra
这一列展示的是额外信息。常见的重要值如下:
候选值 | 含义 |
---|---|
Using index condition |
使用了 ICP(Index Condition Pushed) 优化,也就是在数据存储层取出索引的同时,判断是否可以使用 where 条件过滤,某些情况下可以大幅减少上层SQL 层对记录的索取 |
Using MRR |
使用了 MRR(Multi-Range Read) 优化,也就是在查询辅助索引时,将得到的结果按照主键进行排序,然后按照主键排序的顺序进行数据查找,从而将随机访问转化为较为顺序的数据访问 |
distinct |
一旦 mysql 找到了与行相联合匹配的行,就不再搜索了 |
Not exists |
mysql 优化了LEFT JOIN ,一旦它找到了匹配LEFT JOIN 标准的行,就不再搜索了 |
Using index |
这发生在对表的请求列都是同一索引的部分的时候,返回的列数据只使用了索引中的信息,而没有再去访问表中的行记录,也就是覆盖索引 ,是性能高的表现 |
Using where |
mysql 服务器将在存储引擎检索行后再进行过滤。就是先读取整行数据,再按 where 条件进行检查,符合就留下,不符合就丢弃 |
Using temporary |
mysql 需要创建一张临时表来处理查询,常见于 group by order by 。出现这种情况一般是要进行优化的,首先是想到用索引来优化 |
Using filesort |
mysql 会对结果使用一个外部索引排序,而不是按索引次序从表里读取行。此时mysql会根据联接类型浏览所有符合条件的记录,并保存排序关键字和行指针,然后排序关键字并按顺序检索行信息。这种情况下一般也是要考虑使用索引来优化的 |
Range checked for each Record(index map:N) |
没有找到理想的索引,因此对于从前面表中来的每一个行组合,mysql检查使用哪个索引,并用它来从表中返回行。这是使用索引的最慢的连接之一 |
Using join buffer |
强调了在获取连接条件时没有使用索引,并且需要连接缓冲区来存储中间结果。如果出现了这个值,那么根据查询的具体情况可能需要添加索引来改进性能 |
对表进行优化
索引创建原则
- 1).针对于数据量较大,且查询比较频繁的表建立索引。 单表超过10万数据(增加用户体验) 。
- 2).针对于常作为查询条件(where)、排序(orderby)、分组(groupby)操作的字段建立索引。
- 3).尽量选择区分度高的列作为索引,尽量建立唯一索引,区分度越高,使用索引的效率越高。
- 4).如果是字符串类型的字段,字段的长度较长,可以针对于字段的特点,建立前缀索引。
- 5).尽量使用联合索引,减少单列索引,查询时,联合索引很多时候可以覆盖索引,节省存储空间,避免回表,提高查询效率。
- 6).要控制索引的数量,索引并不是多多益善,索引越多,维护索引引结构的代价也就越大,会影响增删改的效率。
- 7).如果索引列不能存储NULL值,请在创建表时使用NOTNULL约束它。当优化器知道每列是否包含NULL值时,它可以更好地确定哪个索引最有效地用于查询。
表目前的状态,可以看到是没有任何索引的。
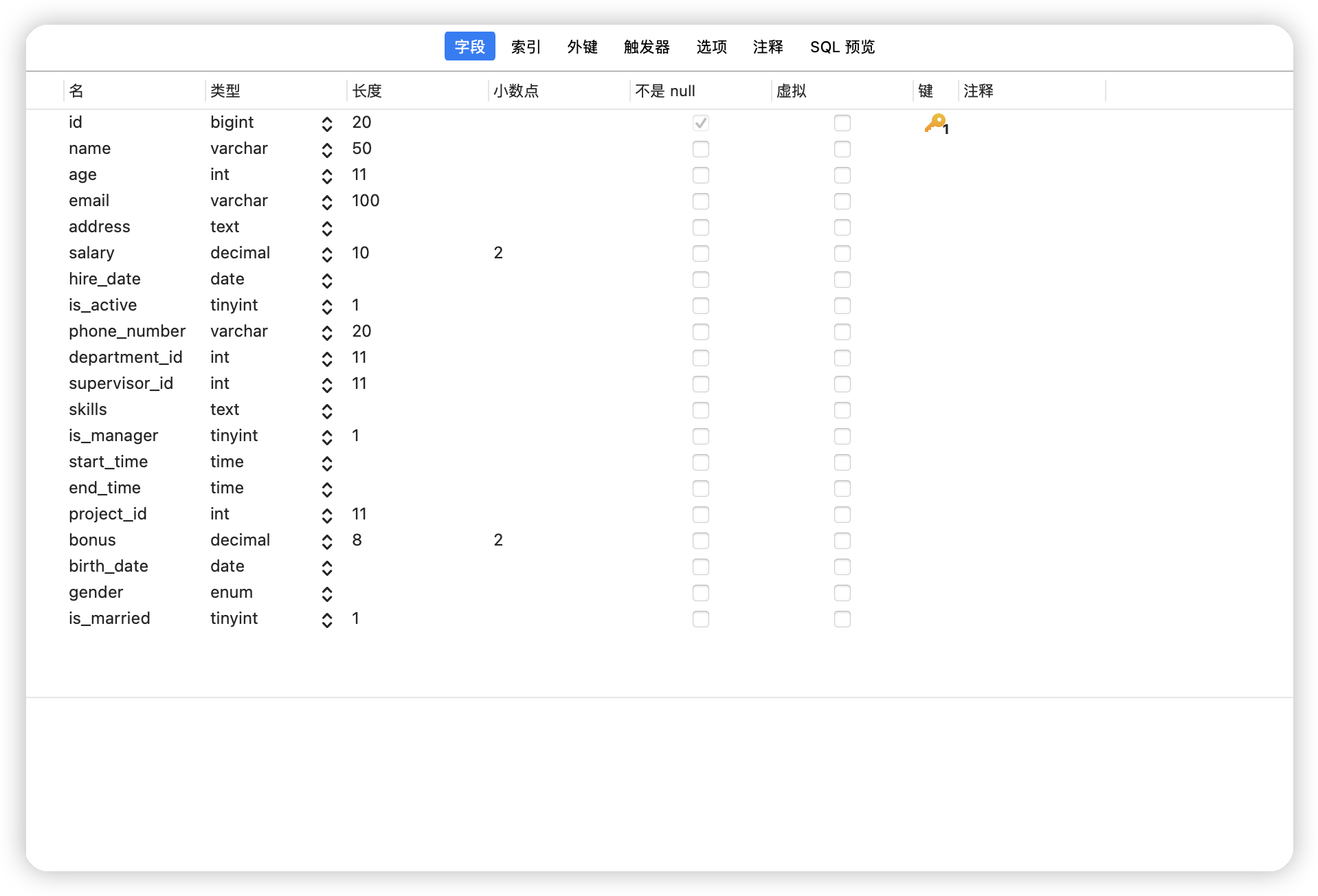
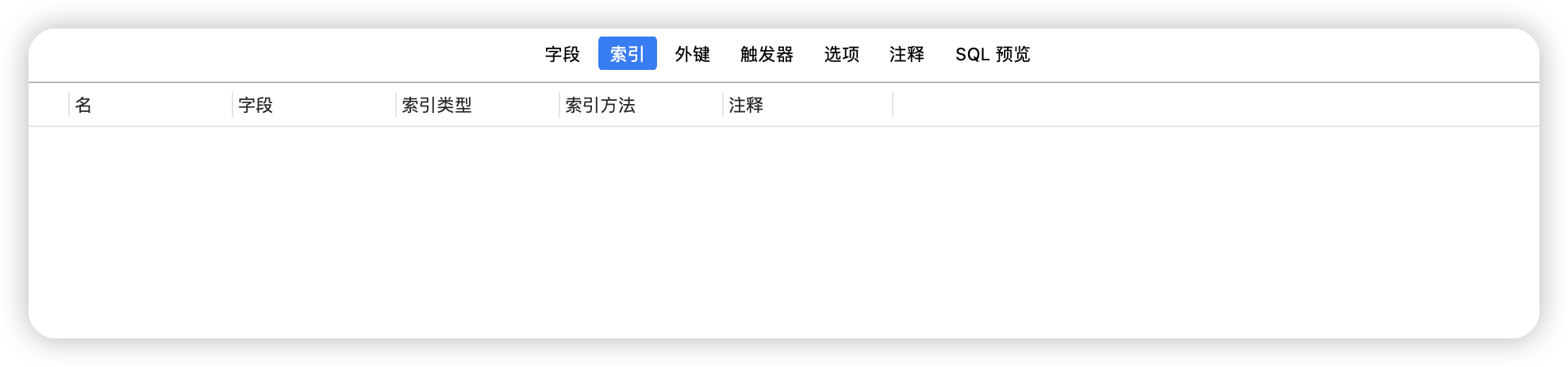
单列索引
主键索引
MySQL 主键索引是一种特殊类型的索引,用于唯一标识数据库表中的每一行数据。主键索引具有以下特点:
- 唯一性:主键索引确保表中的每一行都具有唯一的主键值,这意味着不能有两行数据具有相同的主键值。
- 必须非空:主键字段的值不能为NULL,因为主键的目的是唯一标识每一行数据,NULL 值无法唯一标识。
- 加速检索:主键索引不仅确保数据的唯一性,还可以加速根据主键值进行检索的操作,包括SELECT、UPDATE 和 DELETE 等。
- 默认情况下,MySQL 会为每个具有主键约束的表自动创建主键索引。
在创建主键索引时,你可以选择使用以下方式之一来定义主键:
- 单一列主键:在表中选择一个列作为主键,通常是一个唯一的标识符列,如一个自增长的整数列。
sqlCopy code
CREATE TABLE mytable (
id INT AUTO_INCREMENT PRIMARY KEY,
name VARCHAR(255)
);
- 复合主键:可以将多个列组合起来作为复合主键,这样的主键值由这些列的值组合而成。
sqlCopy code
CREATE TABLE mytable (
column1 INT,
column2 INT,
PRIMARY KEY (column1, column2)
);
在使用主键索引时,你可以更快地检索、更新和删除特定行,因为数据库引擎会使用主键索引来定位数据行。因此,在设计数据库表时,选择适当的主键非常重要,它应该是唯一的且能够明确标识每一行数据。
唯一索引
使用email字段作为唯一索引
未添加索引前
local_test> select email from large_table where email = 'EMOBvwUSJq@example.com' [2023-09-19 20:34:33] 在 1 s 970 ms (execution: 1 s 955 ms, fetching: 15 ms) 内检索到从 1 开始的 1 行 local_test> select email from large_table where email = 'EMOBvwUSJq@example.com' [2023-09-19 20:34:37] 在 1 s 930 ms (execution: 1 s 918 ms, fetching: 12 ms) 内检索到从 1 开始的 1 行 local_test> select email from large_table where email = 'EMOBvwUSJq@example.com' [2023-09-19 20:34:40] 在 1 s 919 ms (execution: 1 s 906 ms, fetching: 13 ms) 内检索到从 1 开始的 1 行
Explain分析
id select_type table partitions type possible_keys key key_len ref rows filtered Extra 1 SIMPLE large_table null ALL null null null null 9891258 10 Using where 添加索引
CREATE UNIQUE INDEX unique_email_index ON large_table (email);
添加索引后
local_test> select email from large_table where email = 'EMOBvwUSJq@example.com' [2023-09-19 20:36:37] 在 13 ms (execution: 5 ms, fetching: 8 ms) 内检索到从 1 开始的 1 行 local_test> select email from large_table where email = 'EMOBvwUSJq@example.com' [2023-09-19 20:36:50] 在 12 ms (execution: 5 ms, fetching: 7 ms) 内检索到从 1 开始的 1 行 local_test> select email from large_table where email = 'EMOBvwUSJq@example.com' [2023-09-19 20:36:51] 在 16 ms (execution: 6 ms, fetching: 10 ms) 内检索到从 1 开始的 1 行
Explain分析,命中唯一索引
unique\_email\_index
id select_type table partitions type possible_keys key key_len ref rows filtered Extra 1 SIMPLE large_table null const unique_email_index unique_email_index 303 const 1 100 Using index 优化了多少倍,1s930ms到13ms,151.54差不多150倍,质的飞跃。
普通索引
针对age字段进行优化,为age添加索引
未添加索引前
local_test> SELECT large_table.age FROM large_table WHERE age < 30 [2023-09-19 19:58:52] 在 6 s 810 ms (execution: 24 ms, fetching: 6 s 786 ms) 内检索到从 1 开始的 2,790,055 行
使用explain进行sql语句分析
id select_type table partitions type possible_keys key key_len ref rows filtered Extra 1 SIMPLE large_table null ALL null null null null 10004321 33.33 Using where 添加索引
CREATE INDEX idx_age ON large_table (age);
添加索引后
local_test> SELECT large_table.age FROM large_table WHERE age < 30 [2023-09-19 20:01:23] 在 5 s 879 ms (execution: 8 ms, fetching: 5 s 871 ms) 内检索到从 1 开始的 2,790,055 行
使用explain进行分析
key
命中idx\_age
索引type
变为了rangeid select_type table partitions type possible_keys key key_len ref rows filtered Extra 1 SIMPLE large_table null range idx_age idx_age 5 null 5002160 100 Using where; Using index 我们看到时间由6.8s优化到了5.8s,提升了1s,所以建议使用联合索引,在海量数据面前,当字段数据区分度不高时,速度提升还是不够明显的。
联合索引
对
age, supervisor_id, is_manager, salary
这四个字段添加联合索引未添加索引前
local_test> select age, supervisor_id, is_manager, salary from large_table where age = 41 and supervisor_id = 20 and is_manager = 1 and salary = 4238.73 [2023-09-19 22:03:31] 在 3 s 154 ms (execution: 3 s 139 ms, fetching: 15 ms) 内检索到从 1 开始的 1 行 local_test> select age, supervisor_id, is_manager, salary from large_table where age = 41 and supervisor_id = 20 and is_manager = 1 [2023-09-19 22:03:33] 在 2 s 354 ms (execution: 1 s 703 ms, fetching: 651 ms) 内检索到从 1 开始的 1,149 行 local_test> select age, supervisor_id, is_manager, salary from large_table where age = 41 and supervisor_id = 20 [2023-09-19 22:03:35] 在 2 s 264 ms (execution: 800 ms, fetching: 1 s 464 ms) 内检索到从 1 开始的 2,303 行 local_test> select age, supervisor_id, is_manager, salary from large_table where age = 41 [2023-09-19 22:03:38] 在 2 s 489 ms (execution: 9 ms, fetching: 2 s 480 ms) 内检索到从 1 开始的 233,300 行
Explain分析
id select_type table partitions type possible_keys key key_len ref rows filtered Extra 1 SIMPLE large_table null ALL null null null null 9891258 0.01 Using where id select_type table partitions type possible_keys key key_len ref rows filtered Extra 1 SIMPLE large_table null ALL null null null null 9891258 0.1 Using where id select_type table partitions type possible_keys key key_len ref rows filtered Extra 1 SIMPLE large_table null ALL null null null null 9891258 1 Using where id select_type table partitions type possible_keys key key_len ref rows filtered Extra 1 SIMPLE large_table null ALL null null null null 9891258 10 Using where 添加索引
CREATE INDEX idx_age_supervisor_manager_salary ON large_table (age, supervisor_id, is_manager, salary);
添加索引后
local_test> select age, supervisor_id, is_manager, salary from large_table where age = 41 and supervisor_id = 20 and is_manager = 1 and salary = 4238.73 [2023-09-19 22:05:47] 在 20 ms (execution: 5 ms, fetching: 15 ms) 内检索到从 1 开始的 1 行 local_test> select age, supervisor_id, is_manager, salary from large_table where age = 41 and supervisor_id = 20 and is_manager = 1 [2023-09-19 22:05:47] 在 20 ms (execution: 6 ms, fetching: 14 ms) 内检索到从 1 开始的 1,149 行 local_test> select age, supervisor_id, is_manager, salary from large_table where age = 41 and supervisor_id = 20 [2023-09-19 22:05:47] 在 28 ms (execution: 6 ms, fetching: 22 ms) 内检索到从 1 开始的 2,303 行 local_test> select age, supervisor_id, is_manager, salary from large_table where age = 41 [2023-09-19 22:05:48] 在 840 ms (execution: 6 ms, fetching: 834 ms) 内检索到从 1 开始的 233,300 行
Explain分析
id select_type table partitions type possible_keys key key_len ref rows filtered Extra 1 SIMPLE large_table null ref idx_age_supervisor_manager_salary idx_age_supervisor_manager_salary 17 const,const,const,const 1 100 Using index id select_type table partitions type possible_keys key key_len ref rows filtered Extra 1 SIMPLE large_table null ref idx_age_supervisor_manager_salary idx_age_supervisor_manager_salary 11 const,const,const 1149 100 Using index id select_type table partitions type possible_keys key key_len ref rows filtered Extra 1 SIMPLE large_table null ref idx_age_supervisor_manager_salary idx_age_supervisor_manager_salary 9 const,const 2303 100 Using index id select_type table partitions type possible_keys key key_len ref rows filtered Extra 1 SIMPLE large_table null ref idx_age_supervisor_manager_salary idx_age_supervisor_manager_salary 4 const 478910 100 Using index 观察到,虽然每条sql都使用到了索引,但是占用有效字段越多,速度提升越明显。
索引失效问题
- 函数操作:如果在查询条件中对索引列进行函数操作,例如使用
CONCAT
、UPPER
、LOWER
等函数,索引可能会失效,因为函数操作会改变列的值,使得无法直接利用索引。 - 数据类型不匹配:如果查询条件中的数据类型与索引列的数据类型不匹配,索引可能会失效。例如,如果索引列是整数,但查询条件中使用了字符串,索引可能不会被使用。
- 不适当的使用
OR
条件:在查询条件中使用OR
条件时,如果每个条件涉及不同的列,并且这些列没有合适的联合索引,索引可能会失效。这是因为索引通常只能有效地用于筛选与一个列相关的条件。 - 低选择性的列:如果索引列的选择性很低,即不同的值很少,那么优化器可能不会选择使用索引,因为全表扫描可能更快。
- 索引列的数据分布不均匀:如果索引列的数据分布不均匀,例如某些值的频率非常高,而其他值的频率非常低,那么索引可能会失效,因为优化器可能认为全表扫描更有效。
- 使用
NOT
条件:在某些情况下,使用NOT
条件可能导致索引失效。优化器可能会难以有效使用索引来处理NOT
条件。 - 小表优化:对于非常小的表,优化器可能会选择执行全表扫描,而不是使用索引,因为全表扫描可能更快。
- 索引碎片:索引碎片化可能会降低索引的性能。定期维护和重建索引可以帮助避免这种情况。
要避免索引失效,通常需要仔细设计表和索引,确保查询条件与索引的列匹配,并避免上述情况。此外,使用数据库的性能分析工具可以帮助你了解查询的执行计划,以便更好地优化查询和索引。
最左前缀
顾名思义:最左优先,以最左边的为起点任何连续的索引都能匹配上。同时遇到范围查询(>、<、between、like)就会停止匹配。
例如:如果建立(a,b)顺序的索引,我们的条件只有b=xxx,是匹配不到(a,b)索引的;但是如果查询条件是a = 1 and b = 2或者b=2 and a=1就可以,因为优化器会自动调整a,b的顺序,并不需要严格按照索引的顺序来;再比如a = 1 and b = 2 and c > 3 and d = 4 如果建立(a,b,c,d)顺序的索引,d是用不到索引的,因为c字段是一个范围查询,它之后的字段会停止匹配
最左前缀原则是数据库索引优化的一个基本概念,它指导了如何有效使用多列索引(联合索引)来加速查询。根据最左前缀原则,索引将首先按照索引列的顺序从左到右使用,只有当查询条件涵盖了索引的前缀列时,索引才能被充分利用。
具体来说,以下是最左前缀原则的要点:
- 索引列顺序:在一个联合索引中,索引列的顺序非常重要。查询条件中的列需要与索引的前缀列的顺序一致,以充分利用索引。这意味着如果索引是
(column1, column2, column3)
,那么查询条件应该首先涵盖column1
,然后是column1
和column2
,最后是column1
、column2
和column3
。 - 左边列优先:最左前缀原则要求索引中的左边列首先出现在查询条件中。如果你只使用索引中的右边列或不连续的列,索引可能无法有效使用。
- 索引覆盖:如果查询只选择了索引中的列,并且没有选择表中的其他列,这被称为索引覆盖(Index Covering),它通常可以提供更高的查询性能,因为不需要访问实际数据行。
- 最佳匹配:索引会根据查询的最左前缀条件筛选数据,所以在索引列中包含了更多条件的情况下,索引效果更好。如果只有一部分查询条件与索引匹配,索引的效率可能会受到影响。
我们在分析这条语句的时候,发现还是命中了索引,这是因为mysql优化器会自动调整查询顺序
explain select age, supervisor_id, is_manager, salary from large_table where salary = 4238.73 and is_manager = 1 and supervisor_id = 20 and age = 41 ;
id | select_type | table | partitions | type | possible_keys | key | key_len | ref | rows | filtered | Extra |
---|---|---|---|---|---|---|---|---|---|---|---|
1 | SIMPLE | large_table | null | ref | idx_age_supervisor_manager_salary | idx_age_supervisor_manager_salary | 17 | const,const,const,const | 1 | 100 | Using index |
当条件变为范围查询 type=range
,查询时间变长,索引匹配失败
local_test> SELECT age, supervisor_id, is_manager, salary
FROM large_table
WHERE age < 30 AND supervisor_id > 20 AND is_manager = 0 AND salary < 3967.55
[2023-09-19 22:11:54] 在 2 s 879 ms (execution: 42 ms, fetching: 2 s 837 ms) 内检索到从 1 开始的 828,718 行
id | select_type | table | partitions | type | possible_keys | key | key_len | ref | rows | filtered | Extra |
---|---|---|---|---|---|---|---|---|---|---|---|
1 | SIMPLE | large_table | null | range | idx_age_supervisor_manager_salary | idx_age_supervisor_manager_salary | 4 | null | 4945629 | 1.11 | Using where; Using index |
- Post link: https://yanxiang.wang/mysql%E5%8D%83%E4%B8%87%E6%95%B0%E6%8D%AE%E7%B4%A2%E5%BC%95%E4%BC%98%E5%8C%96/
- Copyright Notice: All articles in this blog are licensed under unless otherwise stated.